Ron Reid
Group Resource Geologist
Harmony Gold SE Asia Pty Ltd.
This blog post was first published as a series of posts centred around using Leapfrog Software on the orefind.com blog (www.orefind.com/blog) and parts were presented at an AIG talk in Brisbane in 2013. As the last of this series a version of this post titled “Boundary Analysis in Micromine” was published in response to a Micromine Forum question (http://forum.micromine.com/topic/588795-boundary-analysis-plots) asking how to complete a boundary analysis in Micromine.
Most boundary analyses are done using the “down the hole” method where the contact is specified on a drill hole and distances in both directions from the contact counted which can cause errors where the contact is not normal to the drill hole. The better way to do this is using the “Distance from wireframe” method which flags the drill hole or composite file with a distance from a particular wireframe. As the distances from the wireframe are always normal to the surface the actual orientation of the drill hole to the contact doesn’t matter in the analysis. The workflow to do this in Micromine is simple and the whole process can be done entirely within Micromine.
What is Boundary analysis
Boundaries between domains in resource estimation fall into one of four categories (Figure 1);
- Hard – where the estimation within the domain does not see grades outside the domain
- Soft – where the domain boundary is transparent
- Semi-soft – where the boundary is transparent over a short distance (often modelled using an expanded “skin” on the wireframe),
- One way Transparent – this is where the estimate for one domain sees a hard boundary but the estimate for the adjacent domain treats the boundary as transparent. These contacts cause problems statistically due to a “double counting” of metal. How you handle this type of domain requires careful consideration.

Figure 1. The four types of Boundaries possible within an ore deposit, 1 = Hard, 2 = Soft, 3 = Semi-Soft and 4 = a one way boundary (very common in the real world).
Getting the treatment of the boundary wrong can have drastic consequences for an estimate (Figure 2).
- Dragging grade from a high grade domain into a low grade domain when the boundary is hard will artificially increase the grade of the low grade domain.
- Likewise dragging the low grades from the low grade domain into the high grade will drop the grade of the high grade domain.
- Applying a hard boundary to a domain with a soft contact can artificially increase the grade of the high grade domain.
Mostly these affects are only noticeable locally on the boundary of the domain however the influence can be great on projects with small narrow domains, or where the estimator does not appreciate how anisotropic searches can manipulate space and ends up using very large search ranges in the estimate. In all these situations there is the possibility of a significant and material error being built into the resource.
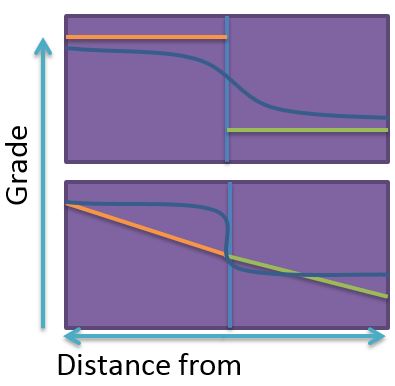
Figure 2. Incorrect handling of the boundary can have drastic consequences for the estimate, Top = using a soft boundary when the boundary should be hard, bottom = using a hard boundary when the boundary should be soft. Dark blue line = estimated grade profile across the boundary (light blue line), green and orange lines represent the actual grade profiles.
These errors can;
- Artificially increase the low grade which may give you enough tonnes to bring a project across the line.
- Conversely an artificially decrease the high grade which could kill a project that may otherwise be a company maker.
Either way if it is wrong you cop the blame. The lesson is “know your boundaries”!!
Boundary analysis is very deposit and model dependant. Several things must be considered prior to commencing any analysis and a couple of rules of thumb I follow for the boundary analysis are;
- Data density – drives your block size and works best if the block is 1/2 to 1/3 your drill hole spacing (arguments over this are in abundance and everyone has their opinion, I’ll take the blue corner…).
- Final block size – your distance bins work best if they are set up to around 1/2 your optimal block size.
- Composite length – the length of your composite should be optimised to your block size and your mining method, I find this process works well if you composite to 1/4 your block size.
Therefore, if we assume our deposit is drilled to 80x80m spacing, a 40x40m block would not be incorrect and following the rules of thumb above our bin spacing would be 20m and our composite length 10m. Of course this is completely subjective and if your deposit is only 8m wide then a 10m composite and a 40m block is likely to be completely wrong for your deposit.
Some practitioners will want to test the boundary at a very detailed level – say 1m in this example. In my opinion this is far too detailed for the level of information we have. If we are estimating into 40m blocks we are doing so because of the spacing of our informing points and we will want to know what the contact looks like at our block size (or half block size). It may appear to be hard at 1m but at the size of the block it is soft – so that is really how it should be treated. If it is actually hard at 1 or 2 blocks distant then the boundary is hard for our purposes.
While a number of programs will do a boundary analysis they are most commonly done using the “down the hole” method of dividing the distances along a drill hole from a specific contact into bins of a set length and then graphing the result. When your drilling is normal or near normal to the contact this is perfectly reasonable and gives good results (Figure 3 and Figure 4). However, generally in the hard rock mining industry this is not the case as many holes will be at a low angle to the contact (Figure 5). This sort of analysis on drill holes that are at a low angle to the contact will always result in a smoothed un-representative graph that causes the contact to appear soft when this is not the case (Figure 6). There are some programs that you can purchase through various consultancies that attempt to measure the distance from a point to a wireframe but having never used these I cannot comment on their accuracy. However, if you have a Micromine licence (or Leapfrog or GoCAD) you have access to a robust and accurate method of conducting a boundary analysis using the “distance from wireframe” method, and the beauty of Micromine is it is all internal – no reason to go external to another program just to complete your analysis.
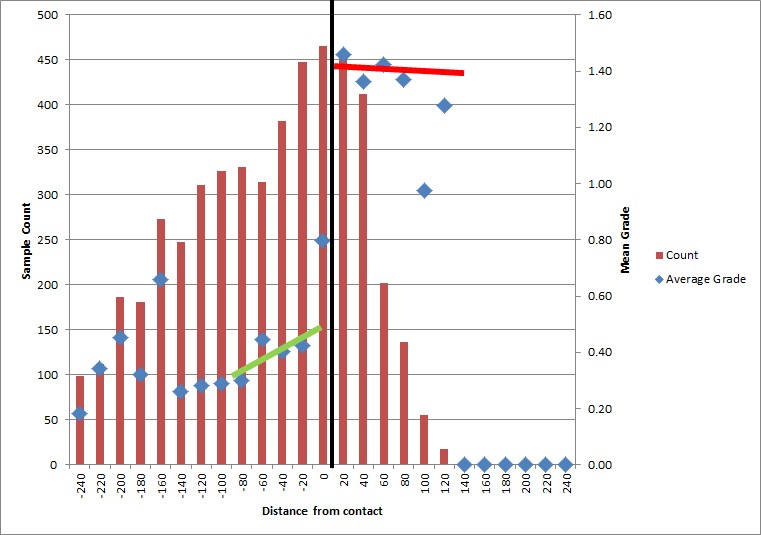
Figure 3. A hard boundary – Top using a standard “down the hole method”, Bottom using the “distance from wireframe” method – the blue diamond at distance 0 is a mixed domain with internal and external data giving you an average of the two (this is not representative), these graphs are from the same contact except the different outputs have resulted in inside and outside being on opposite sides of the graph.
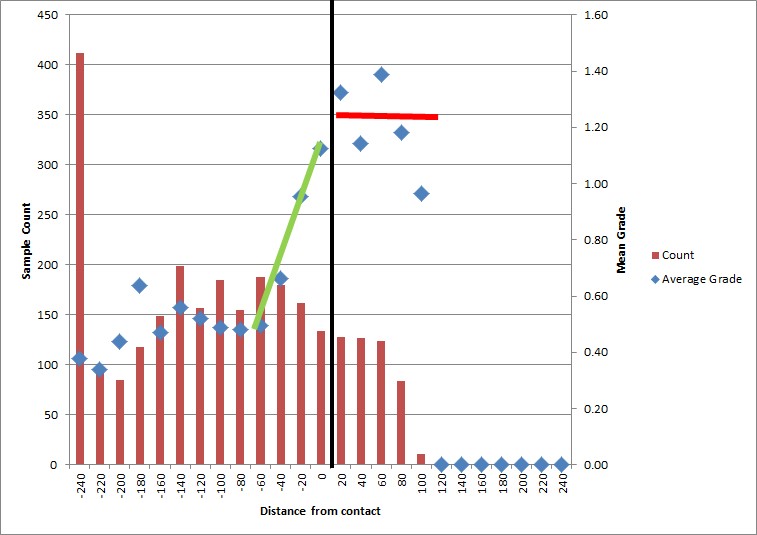
Figure 4. A soft boundary using both methods, Top =down the hole method, Bottom using the distance from wireframe method.
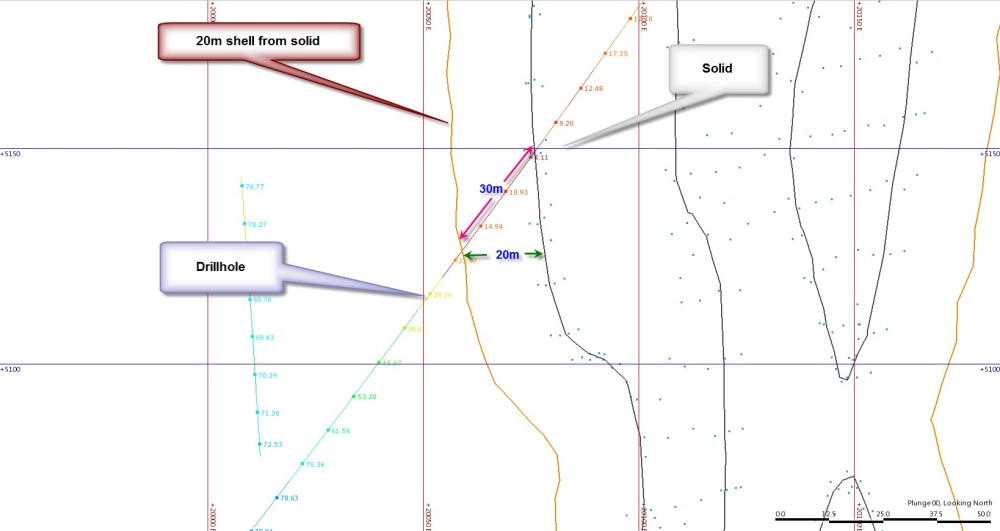
Figure 5. Errors can be built into a “down the hole” analysis through drilling oblique to the contact – distances become “blured” and hide the true nature of the contact.
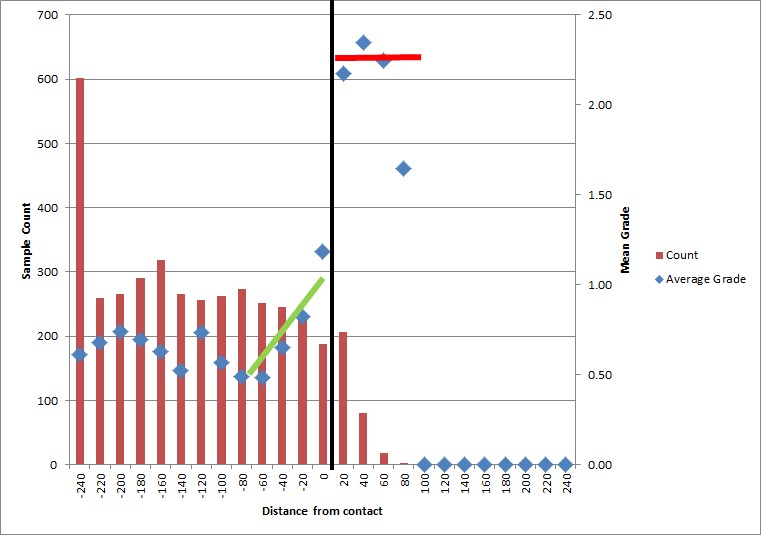
Figure 6. A contact where a large percentage of the holes are at a high angle to the contact. Top a= down the hole method counts a lot of mixed bins resulting in a smooth contact, bottom = when the data is measured as distance from wireframe however you get a very different picture – again these are from the exact same contact – notice the domain is only 100m wide, treating this boundary as transparent had drastic consequences on the grade and economics of this domain.
Mixing of boundaries is another common form of data “pollution” where you may be testing a lithological contact but have neglected to exclude data that may be affecting the result such as an oxide boundary or fault for instance. For any boundary analysis to be accurate it must be done on a like for like basis with as little contamination as possible.
The Micromine Workflow
The first step in Micromine is to have your wireframe you wish to test and a composite file to flag. Remember that boundary analysis can be easily corrupted by improper data selection. For this reason use Micromine’s mesh Boolean options to define a volume with which to flag your data that excludes other contaminating boundaries (Figure 7).
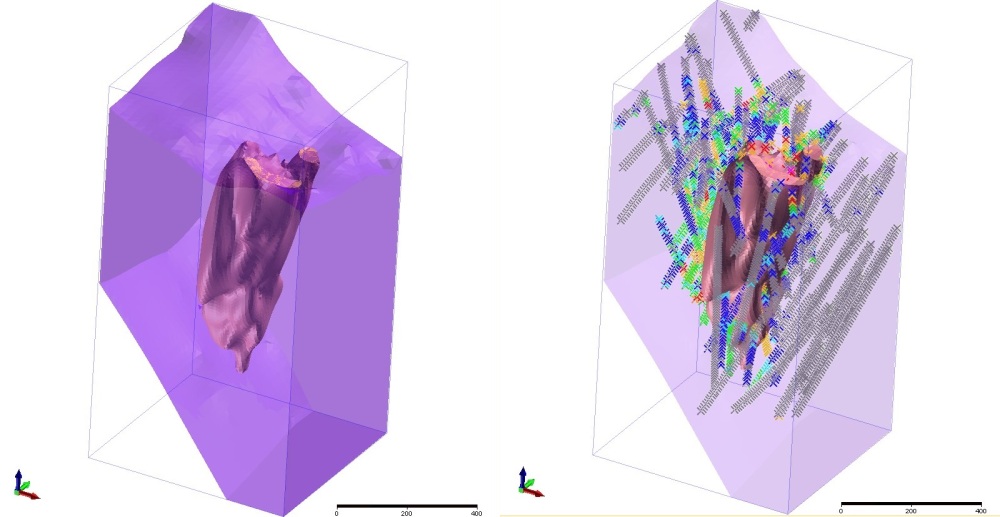
Figure 7. Generate the relevant domain excluding all distracting boundaries – here on the left I have created a volume below the oxide horizon and above a thrust. On the right I have created a subset of the composites that are clipped to the purple domain which I will use to evaluate the distance from the porphyry internal to that domain.
The wireframe I have used here was created using Micromine’s Implicit Modelling process but it could be any implicit or explicitly modelled surface or solid. Note that in Figure 7 there is no data above or below the porphyry that might unduly influence the evaluation. With the data and wireframes in hand the actual workflow is outlined below.
At this point you can flag your composite file with distances from a wireframe using Wireframe -> Calculations -> Distance from Wireframe (Figure 8). There is a lot of information that can be obtained from this form that makes it quite a useful calculation to run. The most important information to record is Distance and Position. The distance is a positive distance between the point and the wireframe surface, it is always positive whether it is from the inside or outside surface. To obtain positive and negative distances you use the position field and code +1 for inside and -1 for outside (or +1 for above and -1 for below when using a surface). For a closed (and valid) solid Outside limits is simply outside the wireframe, for a surface it represents all those samples beyond the extent of the surface – seeing as we have limited our composites to a specific volume if you are using a surface there should be no points beyond the extent of the wireframe and so you need not worry about it. The other options are nice to have and quite useful from a validation point of view – they are the name of the wireframe used (useful when trialling different wireframes – you can easily associate the wireframe to a composite file), the azimuth or direction of the point from the wireframe and the dip of the point from the wireframe surface.

Figure 8. The Distance to wireframes form allows you to flag your composites with distance to wireframe and the position of the point in 3D space with respect to the wireframe volume, use above and below if you are testing a DTM surface.
Once you run the form you have a fully flagged table that you can complete the evaluation in Micromine. First you calculate the positive and negative distances by simply running a calculation on the file to calculate them (File -> Fields -> Calculate), it is a simple calculation of WF_Distance = Distance multiplied by position (Figure 9). This gives you a good way of displaying the data and visually validating the calculation, is it doing what you expect it to (Figure 10)?

Figure 9. A simple field calculation gives us the positive and negative distances needed for the calculations.
You then need to classify the distances into the relevant bins in order to assess the statistics. Because I am looking to run an estimate into 40m blocks using my rules of thumb discussed earlier I have composited to 10m (which also happens to be a multiple of all my sample lengths – good practice), the best bin size to work with in this case is around 20m (half the block size). For this example I am going to classify my data into bins of 20m which will give me 2 “data points” for each block. I can do this using the File -> Fields -> Generate option (Figure 11).
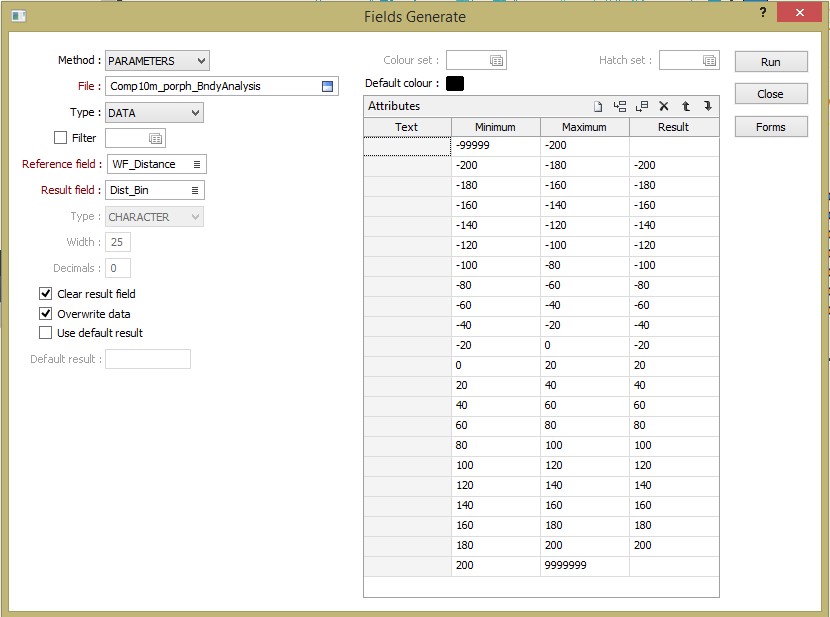
Figure 11. The Fields Generate form – this allows you to classify the distances into Bins for further analysis – here I am using Bins of 20m because my blocks are 40m in size, I leave the bins more than 200m away blank as I am not really interested in this data and at these distances the points start to be influenced by other mineralised bodies and 200m will give me 5 blocks or 10 points of information either side of the contact which should be enough (Yes I know I am clearing then overwriting my results field and I don’t need to do both but “mi nogat wori”…).
This process then flags each sample with a Bin ID, to do this the Wireframe distance field must be numeric – if it is character you cannot select minimum and maximum totals so select to modify the file and check. While you are in the file modify section you have to add a field to the table (call it number/sample number/ Number of Bins – whatever you like as long as it is meaningful) and then fill this with 1 (Thanks to Dave Bartlett from Micromine Support for helping me see this simple solution for a sample count and helping me with the final steps of this process). Then you must sort the file by your new bin field (I sort by the bins first and then WF_Distance second just to be pedantic).
With the composite table flagged by distances, classified into bins and sorted by those bins we can then do a drill hole extract where we extract the data within each bin and average it. If you have a Hole_ID and a depth from and depth to field Micromine will do this extract on a drill hole by drill hole basis when sorted by drill hole and drill hole depth but it still gives us a result we can use, more about this later. If you sort the table by the Bins field first (ascending from negative to positive) the extract will work on the bins only. Go to Drill hole -> Calculations -> Extraction and fill the form as indicated in Figure 12. This will average the assays and sum up the sample count for each bin (and by keeping the file sorted by the HoleID, From and To – each hole if you want that option).
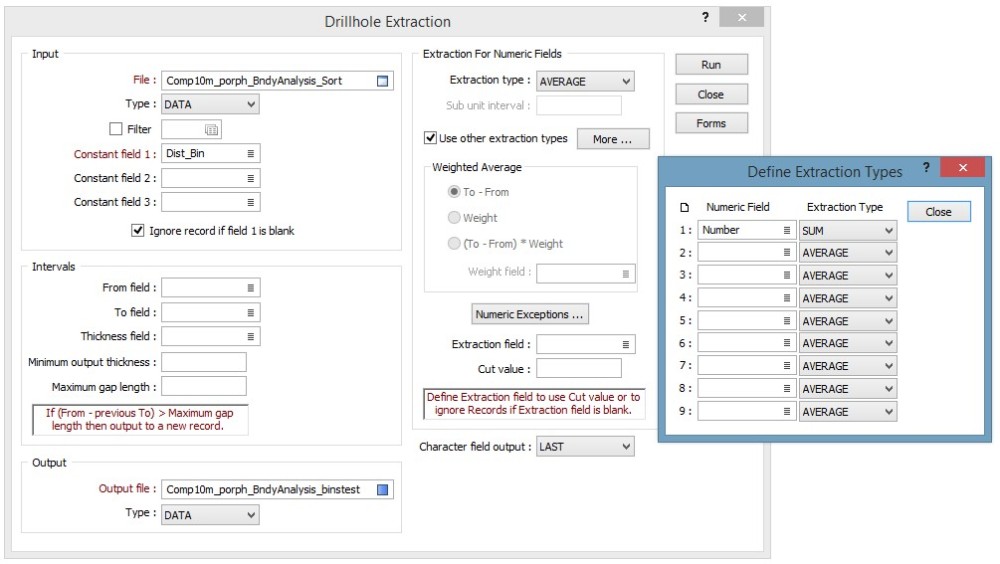
Figure 12. Drillhole Extraction form, my constant field is the distance Bin. I have selected to average the assay fields but I want a count of the number of samples so I need to “use other extraction types” to sum the sample count field I created earlier.
We now have 2 or 3 point files – one complete composite file which is flagged with distance and direction from the wireframe in question which can be graphed, analysed and pulled apart. The information in the main composite file is not only useful for generating the contact analysis but allows you to visually assess what is informing each individual composite and its impact overall. A file that has been composited into distance bins with a sample count for each hole required for the last step in Micromine. And if you have kept the file sorted by HoleID, From and To fields in the composite table prior to running the extract a third file which can also be analysed, pulled apart and assessed, with this table as the bins already done it is an easier process to average the data in each bin using Excel and a pivot table. The pivot table gives you a simple way of grouping the distance data regardless of the Hole ID information, you can then average grades and the sample counts into a format that you can graph (Figure 13), and get a nice tabular representation of the jump in grade if it is there, I prefer to do the lot in Micromine.
There are a number of ways we can analyse all this data solely in Micromine. First of all you can create a basic scatter plot of grade by distance using either the full composited file or the reduced binned by drill hole file, I generally use the full file as this allows more detailed analysis of the data through synced windows but it does have the affect of masking the boundary a tad as it contains a significant amount of data that can deceive the uninformed eye (Figure 14). These sorts of plots however are very good at keeping us honest. When looking at a plot that contains a single point per bin (Figure 13) it is very easy to believe that there is no scatter behind it – seeing such a significant jump in grade in the plot with all the data helps convince you that the final contact analysis is probably correct.
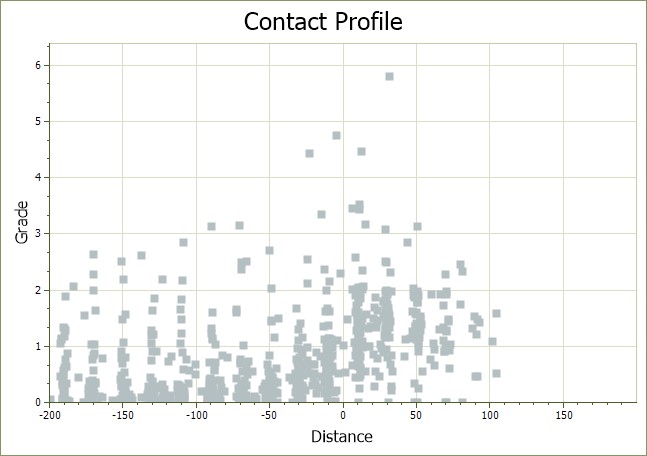
Figure 14. Basic contact profile that plots distance against grade, whilst noisier than a traditional contact profile it does show the sort of scatter you would expect to see in reality and an average line through the data either side of 0m distance indicates that a significant change occurs.
In Figure 15 I have specifically selected one composite, interrogated the dip, azimuth and distance from the wireframe and then created a string file using that information to see what part of the wireframe is informing this point. Very useful when you notice some anomalies in the data and want to find the cause.
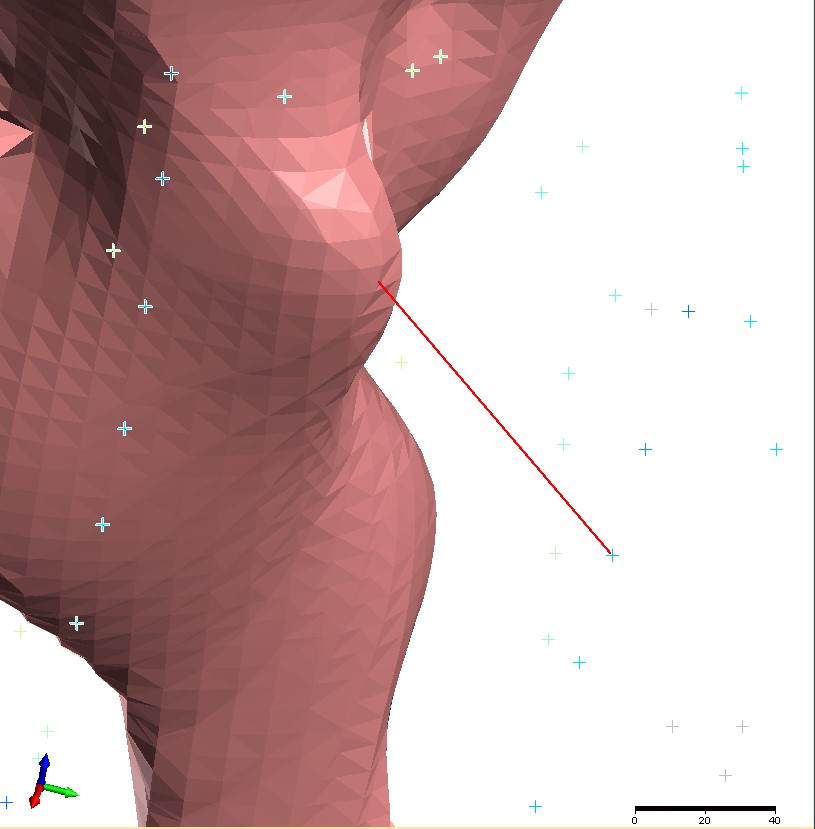
Figure 15. Here I have used the Distance, SurfDip and SurfAzi information in the composite table to identify the exact portion of the wireframe that informs this particular point, remember the information in the comp file is “From” the wireframe so to key in the correct coordinates you must reverse the Azi (+/-180) and Dip angles (90-Dip).
In Figure 16 I have generated some plots from the data and as the various windows have been synced I can click on something in one window and assess the location and impact of this on the analysis. In this case there is an anomalous population of data that sits in the -10 to -30 degree dip window of the histogram, selecting these two bars highlights the data in the stereonet, the main Vizex view and the contact profile plot. I can see that there is a spread of the data both internal to and external to the porphyry wireframe and that while the grades inside the porphyry (Positive distances) are about average for the porphyry the points external to and on the south-eastern side of the porphyry are generally lower in grade than the rest of the dataset which might be important. You could also select all the data above a particular grade and assess the distribution – for instance are all the higher grades in a particular area and do they need to be domained out?
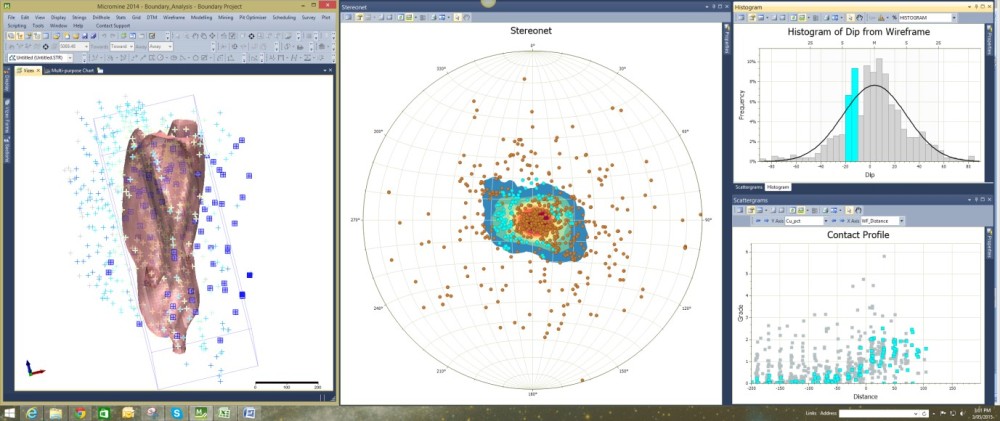
Figure 16. With the various windows linked and the data at hand you can interrogate your data and assess the impact of various populations in the dataset – here for instance there is a prevalence of data in the -10 to -30 degree dip that is biased towards the eastern side. While it does not appear to have an effect on the contact profile analysis internally it does appear to be low on the external dataset.
If you have sorted the composite table by bin prior to running the drill hole extraction you will have a new table where all the information is divided into the relevant distance bins only, with a sample count and averaged grades. With this table there is no need to go external to a spreadsheet program as you can use Micromine’s Multi-Purpose Chart option to graph up the results (Figure 17).
The results are then plotted as a chart that shows the sample count and the average grade per bin. Figure 18 shows the copper with a hard boundary, whereas Figure 19 shows the molybdenum chart, clearly this is a soft boundary with the Moly showing no real change across the contact.
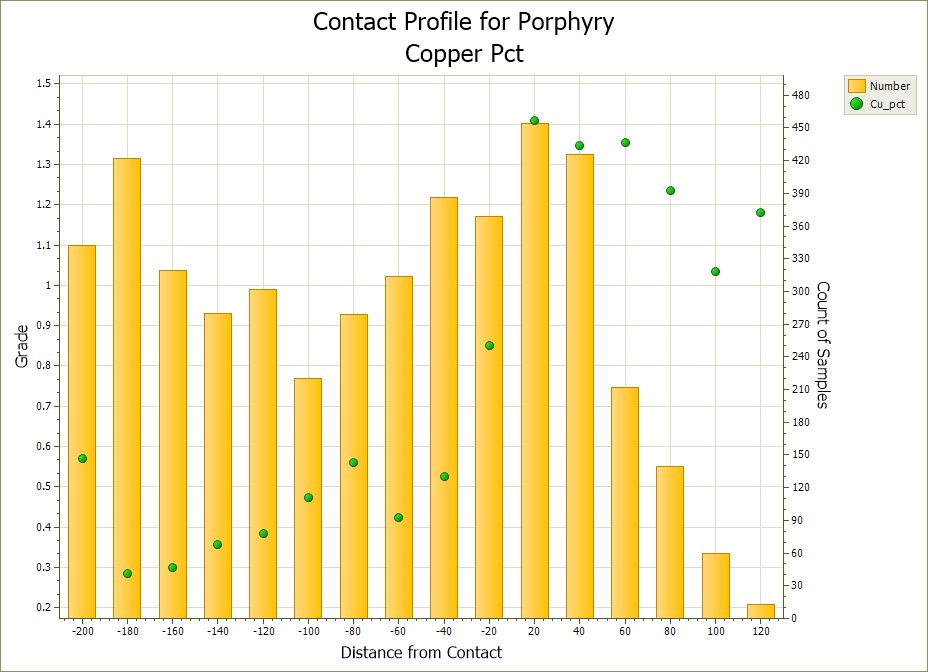
Figure 18. The same contact profile as in Figure 13 but done in Micromine, clearly a hard boundary with over 0.5% jump in copper across the contact.
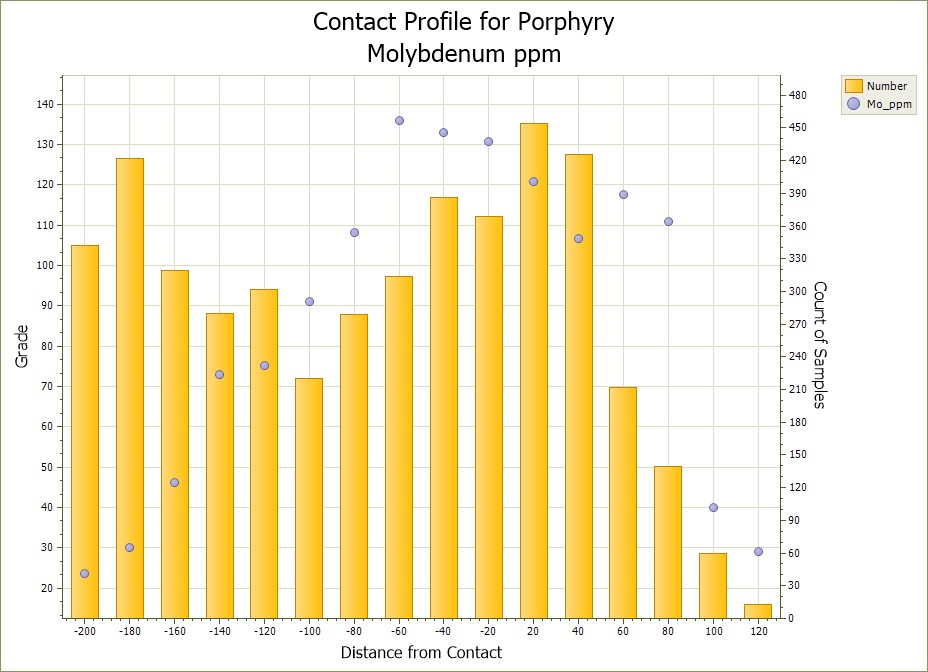
Figure 19. Here we have a Molybdenum chart – clearly here the porphyry contact means next to nothing, just another rock Mo is passing through.
Repeating this analysis over several areas of interest will give a good indication of a realistic search strategy and boundary condition. Large areas can be done rapidly, well within an hour. In this example I have used a thrust and oxidation contact to constrain and assess a lithological contact, the same process can be conducted on any boundary in the dataset, structural boundaries, alteration fronts, oxidation layers etc, very rapidly and with confidence. If your composite file has all your metals of interest to start with then you have everything at hand to rapidly test all your commodities.
Happy Modelling